General Overview of Artificial Intelligence for Interstitial Cystitis in Urology
Article information
Abstract
Our understanding of interstitial cystitis/bladder pain syndrome (IC/BPS) has evolved over time. The diagnosis of IC/BPS is primarily based on symptoms such as urgency, frequency, and bladder or pelvic pain. While the exact causes of IC/BPS remain unclear, it is thought to involve several factors, including abnormalities in the bladder’s urothelium, mast cell degranulation within the bladder, inflammation of the bladder, and altered innervation of the bladder. Treatment options include patient education, dietary and lifestyle modifications, medications, intravesical therapy, and surgical interventions. This review article provides insights into IC/BPS, including aspects of treatment, prognosis prediction, and emerging therapeutic options. Additionally, it explores the application of deep learning for diagnosing major diseases associated with IC/BPS.
INTRODUCTION
Over the years, there has been considerable confusion and evolution in the terminology, definitions, and diagnostic criteria for interstitial cystitis (IC). The definition provided by the International Continence Society is currently the most widely accepted. Bladder pain syndrome (BPS) is characterized by suprapubic pain associated with bladder filling, accompanied by increased daytime and nighttime urination frequency, in the absence of proven urinary infections or other obvious pathological conditions. IC is diagnosed based on clinical symptoms, primarily including urgency, frequent urination, and bladder or pelvic pain. It is characterized by typical bladder abnormalities such as glomerulations or Hunner lesions (HLs), as well as histological evidence of submucosal inflammation and the presence of a certain number of mast cells [1]. To alleviate the confusion surrounding these terms, the ESSIC (European Society for the Study of Interstitial Cystitis) has recently proposed new terminology, diagnostic criteria, and classification [2]. IC is characterized by suprapubic pain related to bladder filling, without any other obvious underlying causes, such as urinary tract infections, and is often accompanied by increased urination frequency and urgency. Multiple treatment options for this condition are actively being investigated [3]. Recently, artificial intelligence (AI) has been integrated into urology research and practice, leading to improved precision in disease diagnosis and the development of predictive models for monitoring responses to medical treatments [4-13]. Specifically, the detection of ureteral strictures and the recognition of urolithiasis have been developed using deep learning and machine learning (ML) techniques [10,12,13].
Researchers have proposed using large multimodal models (LMMs) as a solution to various challenges in the medical field [14]. This review article offers an overview of significant treatment options in urology for IC, emerging therapies, and a summary of diagnostic techniques, prognostic predictions, as well as deep learning or AI-based approaches for the diagnosis, prognosis, and prevention of IC.
RECENT RESEARCH ON BLADDER INTRAVESICAL THERAPY
Various therapeutic approaches have been explored for IC or BPS, including behavioral modifications, medications, intravesical drug instillation therapy, and surgery. Hydrodistension, a commonly employed treatment, provides pain relief for an average duration of 4.4 months. However, the clinical course of IC is marked by periods of improvement and exacerbation. As a result, the primary goal of treatment is to manage symptoms rather than to achieve a complete cure, necessitating patient education for long-term management [3,15]. There is no definitive cure for IC, and symptomatic treatment remains the mainstay. Common therapies include oral medications such as pentosan polysulfate sodium (PPS), amitriptyline, cimetidine, and hydroxyzine, which help protect the bladder lining, as well as intravesical therapies like dimethyl sulfoxide, heparin, and lidocaine. PPS, an oral agent, structurally resembles glycosaminoglycan (GAG) and is thought to aid in the regeneration and supplementation of the GAG layer in the bladder mucosa, thereby reducing its permeability.
PPS is an oral treatment approved by the U.S. Food and Drug Administration (FDA) for the management of IC in both men and women. It works by repairing and protecting the GAG layer of the bladder wall. Research by Parsons et al. [16] and Nickel et al. [17] has demonstrated that PPS therapy leads to symptomatic improvement in IC, with patients in the treatment group experiencing a significant reduction in pain compared to those in the placebo group. However, the length of treatment appears to be more critical than the dosage, as suggested by Nickel et al. [17].
PPS is a commonly prescribed oral treatment for IC, known for its convenience and the lack of pain or risk of infection that can accompany bladder instillations. The typical regimen for PPS involves an initial dosage of 100 mg 3 times daily, starting at 200 mg per day for the first few weeks and then gradually increasing the dose. A standard treatment duration spans 3–6 months to evaluate its effectiveness. Although PPS is generally considered safe, it can cause rare side effects such as liver damage, reduced platelet count, blood clotting issues, gastrointestinal disorders, and hair loss. Despite the advantages of GAG-replenishing agents, oral therapies have inherent limitations. The medication must navigate the digestive system and enter the bloodstream before reaching the bladder, which may diminish its effectiveness and heighten the risk of side effects. Moreover, long-term oral administration of PPS has the potential to cause serious side effects.
In contrast, bladder instillation provides a treatment that is more targeted to the bladder, reducing systemic side effects while ensuring high drug concentrations remain within the bladder itself. A variety of therapies, such as dimethyl sulfoxide (DMSO), bacillus Calmette-Guérin, hyaluronic acid (HA), resiniferatoxin, heparin, chondroitin sulfate, and liposome injections, have been effective in mitigating symptoms of IC by protecting the bladder’s mucosal layer through the administered drugs. These agents offer a range of therapeutic actions, including reducing bladder inflammation, forming a protective barrier on the bladder mucosa to block the infiltration of harmful substances, and shielding the bladder from microdamage.
Intravesical treatment involves the direct administration of drugs into the inflamed bladder, allowing high-dose drug delivery with fewer systemic side effects than oral administration. Studies by Morales et al. [18], Porru et al. [19], and Riedl et al. [20] have reported positive responses to intravesical HA treatment, with notable symptom improvements and minimal side effects. Doctors often employ combination therapies, which use a mix of various substances, to enhance therapeutic outcomes. Ongoing studies are exploring the potential benefits of such combination treatments. For example, Leppilahti et al. [21] observed symptom improvement following weekly bladder instillations of HA, while Ghoniem et al. [22] reported symptom relief in 92% of patients with a combination treatment of DMSO, methylprednisolone, and heparin sulfate. Whitmore [23] achieved positive results with a cocktail treatment, and Davis et al. [24] noted better treatment outcomes in a group that received both oral and bladder instillation of PPS compared to a group that received only oral administration.
NEW RESEARCH ON BLADDER INTRAVESICAL THERAPY
IC, once thought to be a rare condition, is now understood to arise from a variety of contributing factors. Researchers are investigating a potential treatment strategy that employs an advanced ultrasound-guided microbubble-mediated drug delivery system. This approach could provide enhanced and synergistic effects by incorporating multiple drugs.
Recent research papers have introduced the concept of an ultrasound-mediated microbubble drug delivery system. This system involves encapsulating therapeutic drugs within microbubbles, which are then introduced into the bloodstream. The drugs are selectively released to target organs using ultrasound, resulting in localized therapeutic effects. In a specific study, researchers investigated the impact of this drug delivery system on an animal model of orchiectomy and testosterone-induced benign prostatic hyperplasia in rats [25]. The method entails loading microbubbles with therapeutic drugs and administering them intravascularly. Ultrasound is subsequently employed to direct the microbubbles to the desired location, tailored to the disease and the specific drugs needed for treatment [25].
The ultrasound-guided microbubble-mediated drug delivery system effectively enhances the permeability of therapeutic drugs in targeted organs or cells. A range of drugs can be encapsulated within microbubbles, which are customized according to the specific disease being addressed. Additionally, the application of safe ultrasound within the human body offers a significant advantage (Fig. 1). The bladder, which is situated posterior to the pelvic cavity, is readily accessible via ultrasound and is an ideal candidate for targeted drug delivery. The development of an ultrasound-guided microbubble drug delivery system, specifically aimed at the bladder, holds promise for improving the treatment of urinary dysfunction.
Min et al. [25] have developed an ultrasound-guided microbubble-mediated drug delivery system that selectively targets the bladder and prostate, proving effective in representative urological conditions. They conducted experiments to evaluate the stability and delivery efficiency of the system at various ultrasound stimulation intensities, established an ultrasound stimulation protocol, and encapsulated candidate drugs within liposome nanoparticles contained in microbubbles for efficacy analysis. These drugs were then administered to animal models with overactive bladder and prostatic hypertrophy. The results indicated that therapeutic drugs encapsulated in microbubbles are successfully delivered to the intended site when subjected to ultrasound stimulation at a specific intensity, resulting in enhanced therapeutic efficacy. These findings imply that by selecting appropriate drugs for encapsulation in microbubbles and by developing ultrasound protocols, it is possible to create treatments for challenging diseases—especially for conditions that currently lack specific therapeutic approaches and are only symptomatically managed.
Therefore, employing complex drugs, such as PPS and HA, within an ultrasound-induced microbubble-mediated drug delivery system for bladder treatment has the potential to minimize the side effects associated with oral treatments. This approach may yield more efficient therapeutic outcomes, thereby enhancing patient quality of life and advancing treatment technology through the acquisition of proprietary technology. Based on these studies, animal experiments are being prepared for the development of an IC treatment.
APPLICATIONS OF ARTIFICIAL INTELLIGENCE
ML encompasses a range of techniques designed to automatically identify patterns in data and leverage them for predictions or decision support in uncertain scenarios [14,26]. ML falls under the broader category of AI and relies heavily on large datasets with minimal human involvement in the decision-making process. Deep learning, a specific subset of ML, employs artificial neural networks with multiple layers to emulate the intricate human cognitive system, and it is gaining attention for its ability to analyze extensive healthcare data. Although artificial neural networks have existed since the 1950s, their practical application was limited by computing power and data availability. However, recent technological and algorithmic advances have enabled deep learning to achieve impressive results in various fields. ML has been deployed for the detection of structural irregularities, often resulting in computer-aided diagnosis (CAD) systems. However, early CAD systems tended to generate more false positives than human readers, leading to longer assessment times and additional biopsies. With the advent of deep learning, it is anticipated that these limitations can be overcome, thereby enhancing detection accuracy and improving the productivity of human readers. Deep learning is also being applied to extract valuable insights from large medical datasets, such as lesion detection, differential diagnosis suggestions, and preliminary radiology reports. In recent years, deep learning has made significant advances in medical fields, particularly in diagnostic imaging [27-31]. AI research using deep learning models for medical images has shown promising progress in classification, detection, and segmentation (Fig. 2). These are critical areas in AI research, with the continuous development of novel methods across various disciplines.
Several studies have demonstrated that deep learning has surpassed human capabilities in detecting various diseases, including bladder cancer [21-24,28,32-34]. The application of AI [35] enhances the accuracy of disease diagnosis and facilitates the development of predictive models for monitoring the efficacy of medical treatments (Fig. 3) [9,36,37]. Iwaki et al. [8] developed a deep learning-based CAD system to improve cystoscopic identification of HLs. Accurate detection of HLs during cystoscopy is essential for enhancing treatment outcomes in patients with Hunner-type IC. However, this task can be challenging due to the variable appearance of HLs. To improve the accuracy of cystoscopic HL detection, Iwaki and his team processed images from various cystoscope types.
They outlined a region of interest (ROI) in each image, adjusting the color tone and brightness of the bladder mucosa within the ROI. Subsequently, they employed a pretrained convolutional neural network (CNN) model known as InceptionResNetv2 [38], which had been initially trained on natural images from the ImageNet database (http://www.image-net.org), to develop CAD systems. To address the limited number of training images, they implemented a 5-fold cross-validation method for evaluating the CAD systems. The training dataset was randomly partitioned into five subsets, or into N subsets as in jackknife cross-validation. The CNN model was then retrained using images from four of the subsets or N-1 subsets, while the retrained models were validated with images from the remaining subset. In the field of urology, AI algorithms that leverage deep learning have been formulated to identify specific diseases or structures in medical images [10-12].
In addition, various studies [14] have employed generative pre-trained transformer (GPT)-4V or LMMs in the medical field. Medical images, such as x-rays and computed tomography scans, as well as clinical information, frequently display considerable variability due to the diverse demographics of patients and the different imaging equipment used. Furthermore, accurately interpreting the visual content in these images requires a high degree of expertise. The technique for detecting bladder intravesical diseases or complex symptoms using LMMs [14,39], which rely on multimodal deep learning, is crucial for assisting clinicians in medical settings. There is a need to develop and improve various support systems that leverage AI technology.
PROPOSING THERAPY BASED ON PROGNOSTIC PREDICTIONS
In the field of urology, the use of AI in diagnosing diseases and recommending treatments, as well as the creation of personalized diagnostic and therapeutic strategies, is highly significant. It enables the delivery of more accurate and effective prognostic and therapeutic options to patients, especially those with chronic conditions such as IC.
AI, deep learning, and the innovative ultrasound-guided microbubble drug delivery system [25] are pivotal in the field of urology. The composition of urine includes various stimulating elements such as ions, minerals, waste products, and bacteria, with the GAG layer acting as a protective barrier against their penetration into the bladder urothelium. However, in patients with IC, this GAG layer is often compromised. The ultrasoundguided microbubble drug delivery system provides a method for restoring the GAG layer by directly administering drugs that contain components similar to those of the GAG layer into the bladder. This technique is crucial in developing personalized therapeutic strategies for the management and prevention of IC and BPS.
The methodology involves creating a deep learning model, such as an LMM or GPT-4 [14], or GPT-4V, to predict a patient’s prognosis and recommend the most appropriate treatment strategy. GPT-4V’s ability to process combined image-text pairs also allows it to utilize previous medical scans and patient diagnosis histories (Fig. 4), which are critical components of the diagnostic procedures conducted by medical professionals [39]. Given that IC/BPS can present with a wide range of symptoms and progressions, conventional treatment methods may be of limited benefit. Consequently, personalized treatment strategies that consider individual prognoses are essential. This requires the development of a predictive model that evaluates a patient’s prognosis and anticipates the impact of treatment based on various factors, including personal attributes, medical history, and diagnostic outcomes. Therefore, by harnessing the insights from the deep learning-based prediction model or LMM, an individualized treatment plan is formulated for each patient. This involves assessing the expected outcomes of treatment derived from the prediction model’s findings and providing recommendations for appropriate medications or surgical interventions. By offering personalized treatment strategies, this approach has the potential to enhance the efficacy of the treatment.
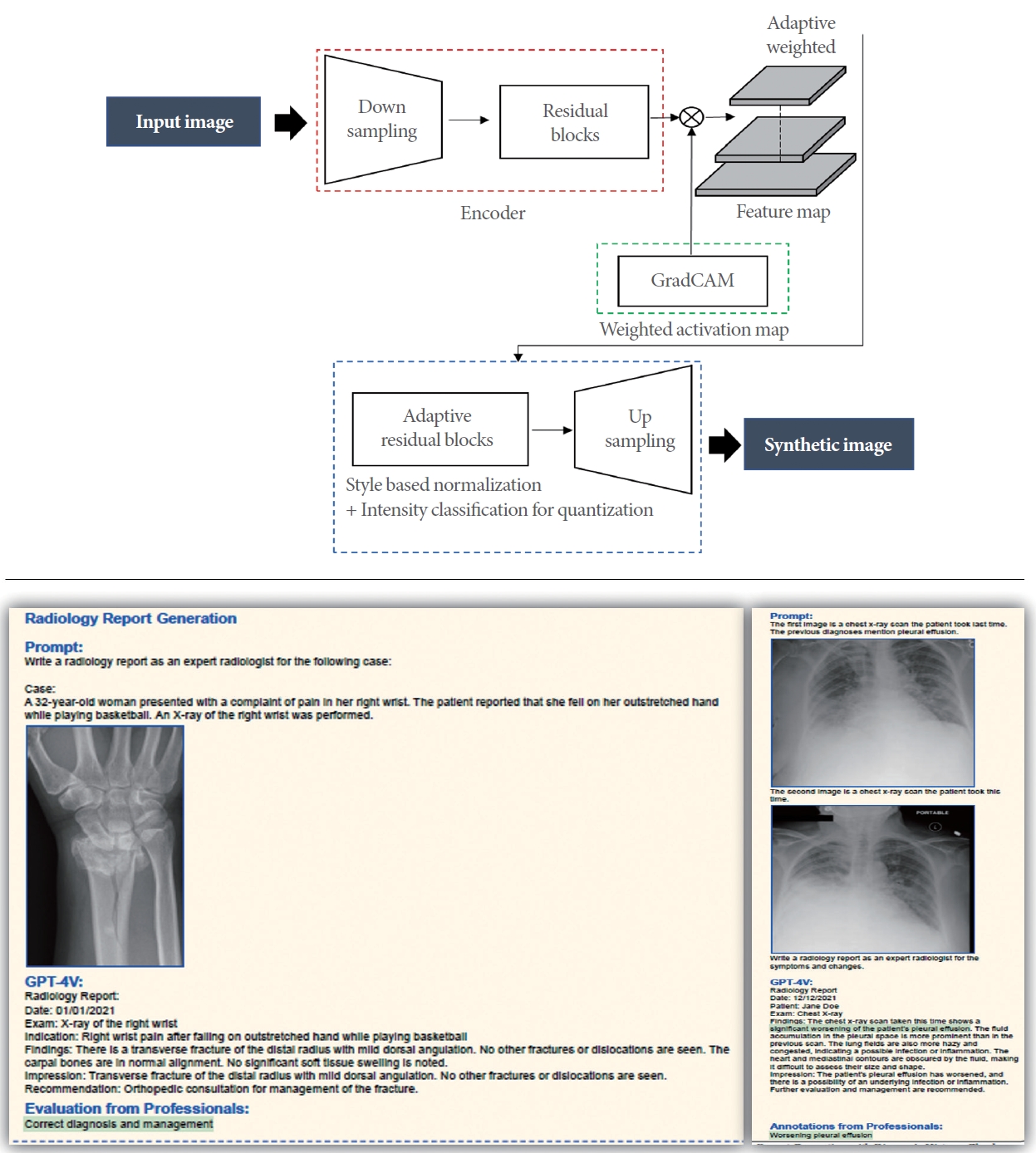
An example of a generative adversarial network (GAN) or generative model. Upper panel: It is to generate synthetic images from real images using GAN. This model is used to generate or transfer images in clinical fields (lower panel). It is the examples using GPT-4V (generative pretrained transformer-4 with vision) in medical fields.
CONCLUSION
This article offers a comprehensive examination of the core technologies employed in diagnosing, treating, and predicting outcomes for IC/BPS, as well as recent advancements in IC treatment and the application of AI in identifying significant diseases associated with IC/BPS. AI systems can be designed to predict patient outcomes and tailor treatments for chronic conditions such as IC. Nevertheless, it is essential to thoughtfully consider the ethical, regulatory, and legal implications associated with using patient clinical data in the development of AI. In conclusion, the advancement of AI and personalized treatment approaches in urology has the potential to be powerful tools for decoding, elucidating, and implementing complex medical data.
Notes
Grant/Fund Support
This work was supported by Chungnam National University.
Conflict of Interest
No potential conflict of interest relevant to this article was reported.
AUTHOR CONTRIBUTION STATEMENT
· Conceptualization: SY
· Formal analysis: YC, PJM, SY
· Methodology: YC
· Writing - original draft: YC, PJM
· Writing - review & editing: YC, PJM, SY
Acknowledgements
We would like to thank the Advanced Medical Imaging Institute in the Department of Radiology at Korea University Anam Hospital in the Republic of Korea, and researchers for providing software, datasets, and various forms of technical support.